The trick with AI isn’t just using it, it’s using it well. Whether you’re a marketer, a business owner, or someone just curious about the hype, the key is to figure out your main goal. What problem are you solving? Applying this to generative AI, like ChatGPT, makes all the difference in how you use it effectively. The clearer you are about what you want, the better your AI responses will be.
If you've ever worked with a CMS, authored blog posts, or managed a website, you're likely familiar with ALT text for SEO purposes. Writing ALT text involves describing an image clearly and concisely for accessibility purposes, ensuring everyone can understand the content. Similarly, crafting a clear AI prompt is crucial for obtaining the best output from AI models. While ALT text makes content accessible to all, AI prompts require precision to trigger specific tasks, whether it’s content generation or data analysis.
For example, if you want an AI tool like Gemini to generate an image for you, be specific. Don’t just say, “Generate an image of a computer.” That’s like asking a chef, “Make me dinner.” What kind of dinner? Spicy, vegetarian, or quick and easy? Be clear about your expectations so the result matches your needs.
For instance:
Vague Prompt: "A computer"
Clear Prompt: "A friendly-looking, anthropomorphic computer with a large monitor for a face, sitting at a desk and smiling, Pixar style"
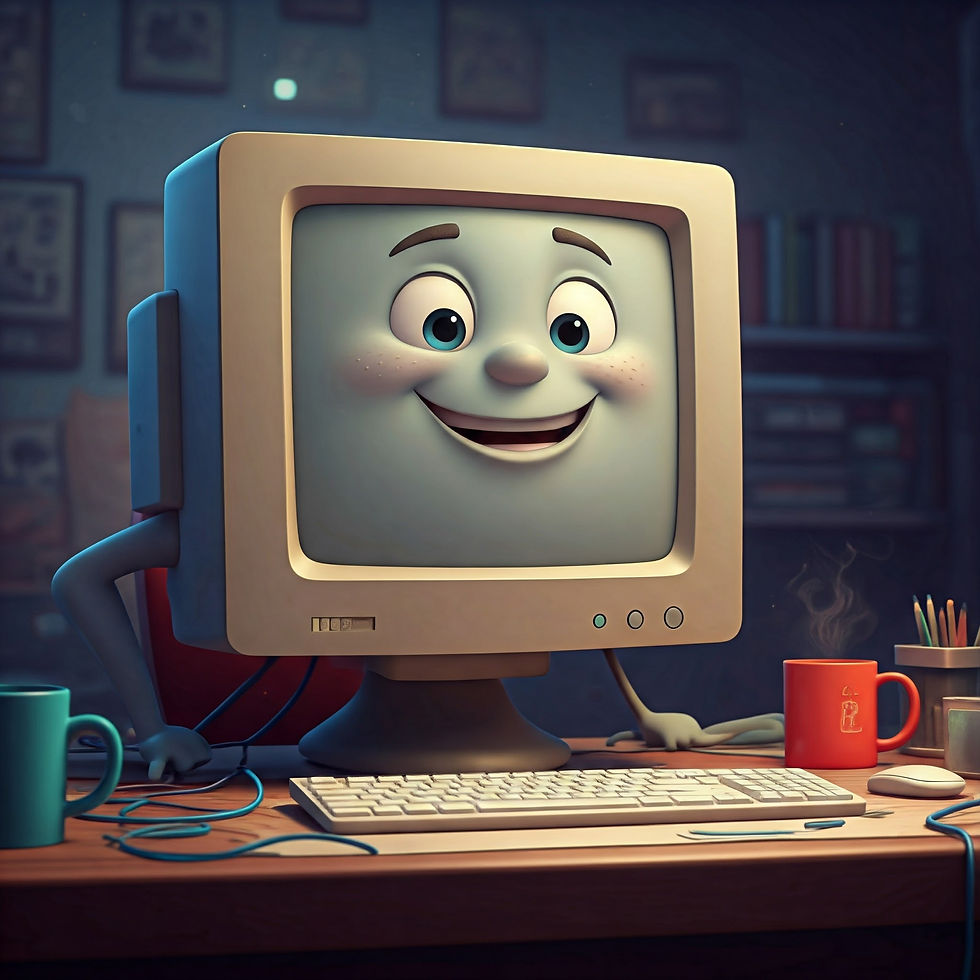
The Bigger Picture
AI isn’t a magic wand. It’s a tool, and like any tool, its value depends on how you use it. As we step into 2025, the question isn’t whether AI will replace jobs or transform industries (spoiler: it will), but how we adapt and evolve alongside it. But there’s one very important thing that we sometimes forget: AI learns from the data we feed it. And guess what? If that data is biased, the AI will be too.
Bias in AI isn’t just an inconvenient flaw; it’s a real danger. It’s the kind of thing that can misrepresent people, cultures, and communities, making AI more of a liability than a tool. If left unchecked, AI can perpetuate harmful stereotypes or make decisions that negatively affect marginalised groups. This is a serious issue, and one that’s gaining increasing attention as AI becomes more embedded in our daily lives.
For example, consider facial recognition technology. Research has shown that certain systems have been less accurate when identifying people of colour, especially women of colour. If AI systems aren’t trained on diverse datasets, the results can be skewed, leading to unfair outcomes, like biased hiring decisions or inaccurate credit scores. One notorious example comes from a study conducted by MIT in 2018, which found that commercial facial recognition systems had significantly higher error rates when identifying darker-skinned women than lighter-skinned men.
This is not just about flawed outputs, it’s about fairness, equity, and trust. AI doesn’t just influence who gets a job or a loan; it can shape our culture, influence how we are perceived, and even determine who gets access to critical services.
More importantly, using AI, whether as an individual or as an organisation, requires ensuring data integrity and privacy throughout the entire AI lifecycle. While we are still mitigating the risks of AI, these risks are becoming increasingly evident. Day by day, we hear and see studies, and research about AI risks, and investments and funding are pouring into AI-powered companies and projects. This makes it essential to not only know how to use AI effectively but also understand how to address its risks and challenges within your sector, role, or business functions. To ensure accuracy and reliability, always double-check and proofread AI-generated content, monitor outputs, and cross-reference with trusted sources. By doing so, AI can become a more effective and dependable tool.
So how do we combat some fo these challenges like AI bias and hallucinations ? Well, here are a few key steps that every AI project should follow throughout its lifecycle:
Diverse Datasets: Make sure the data used to train AI systems is representative of all people and your customers, not just a narrow subset of society. Diversity in data = fairness in output.
Constant Monitoring: AI models need continuous checking to identify and address any biases that may emerge over time. It’s not a “set it and forget it” situation.
Transparency: Companies should be open about how AI is used and how decisions are made. If AI is used in hiring, for example, job seekers should know how it works and what data it relies on.
Human Oversight: While AI can handle a lot of tasks, we still need humans in the loop to ensure that any potential biases or errors are caught before they cause harm.
At the end of the day, AI is only as good as the humans who create it. If we're not careful, AI could reinforce existing inequalities, instead of helping us break them down. So, let’s make sure we’re teaching our AI systems to be fair, kind, and respectful of all the people they represent.
The good news? You don’t need to be a tech wizard to start. The first step is understanding what AI can do for you and taking it from there.
Here’s to a year of embracing the future, one smart decision at a time.
Comments